
NEWS
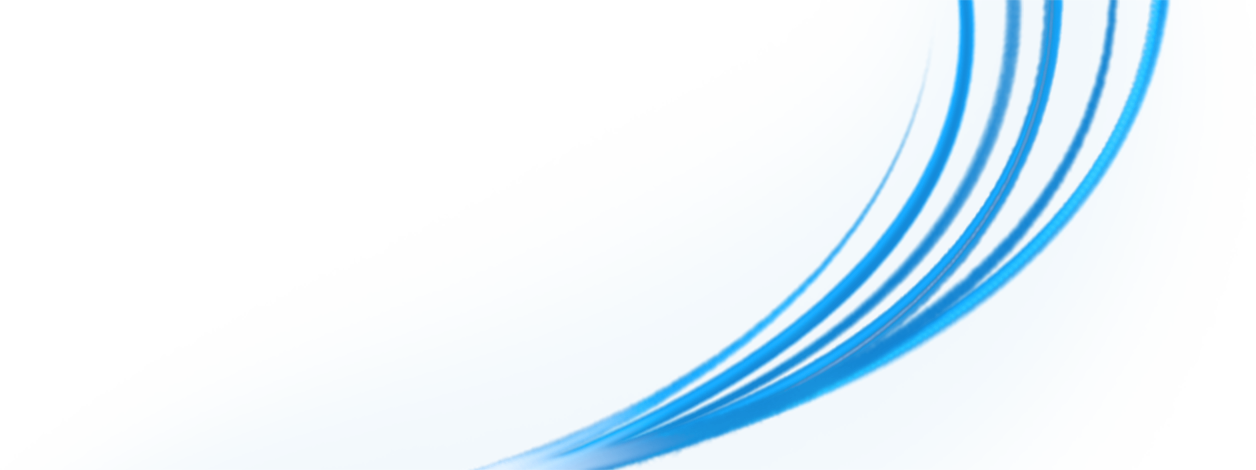
bp and ORCA Computing Team Up to Explore Quantum-Powered Innovation in Computational Chemistry
by William Clements (ORCA Computing), Corneliu Buda (bp), Claudia Perry (bp), Peter Lemke (bp)
Unveiling Quantum Computing’s Promise in Computational Chemistry
Quantum computing has generated excitement and attention as a revolutionary technology capable of overcoming many limitations of classical (i.e. non-quantum) high performance computing (HPC). While the overall performance and size of HPC systems continues to grow, many individual applications are experiencing performance slowdowns, and the energy consumption of systems is becoming unsustainable. Breaking through these limitations and accelerating computationally complex research challenges in chemistry and other fields motivated this collaboration to explore the potential of quantum.
Exploring Quantum Acceleration in Computational Chemistry
Investigating molecular structures is an important pursuit in computational chemistry, especially in fields likes biofuel formulation, material innovation, and pharmaceutical development where research acceleration is critical. The specific problem considered here is significant across the energy industry, as molecule’s possible structures directly determine many of its physical and chemical traits. However, the vast array of possible configurations and high computational requirements make it difficult for traditional methods to find low-energy conformations for certain molecules.
To address this issue, bp and ORCA partnered to explore a hybrid quantum-classical approach using generative adversarial network (GAN) algorithms. This approach aims to generate low-energy conformations of small and medium size hydrocarbon molecules, offering a potential solution to the computational hurdles faced in molecular exploration.
The Hybrid Approach: Quantum-Classical Collaboration
Although large-scale universal quantum computers are still several years away, recent advances in hardware have led to the development and commercialization of small-scale quantum processors that will solve specific problems much faster than classical computers. The best way to explore and leverage the new computational abilities provided by near-term quantum processors is within hybrid workflows involving both a quantum processor and a classical HPC system. The application is divided up into tasks that can be accelerated with a quantum processor and into tasks that are best left to the HPC system. Coordination between these tasks is achieved at both hardware and software levels across both system types.
Bridging Quantum Computing with Chemistry: The Exploration
To explore how quantum impacts computational chemistry, experts at bp and ORCA used a hybrid quantum/classical machine learning approach to generative modelling to produce conformations of molecules. The multidisciplinary team was composed of experts in quantum computing, quantum information science, machine learning and chemistry. The team utilized a GAN (Generative Adversarial Network) to train a hybrid quantum/classical generator. This generator utilized a photonic quantum processor and a GPU to analyse a dataset of alkane molecules, creating conformers with a specified energy. The first validation of the approach was to determine that molecules with the precise energy were generated. Not only was this demonstrated, but the hybrid generator significantly outperformed an equivalent classical generator at this task. This exploratory work shows that near-term quantum processors hold potential for improving the performance of machine learning algorithms in chemistry.
Towards the Future: Optimism and Implementation
With the rapid improvements in the performance of quantum processors, there is optimism that, in the near future, this approach could be extended to other applications and implemented in production computing infrastructures, greatly benefiting researchers.