
REPORTS & PUBLICATIONS
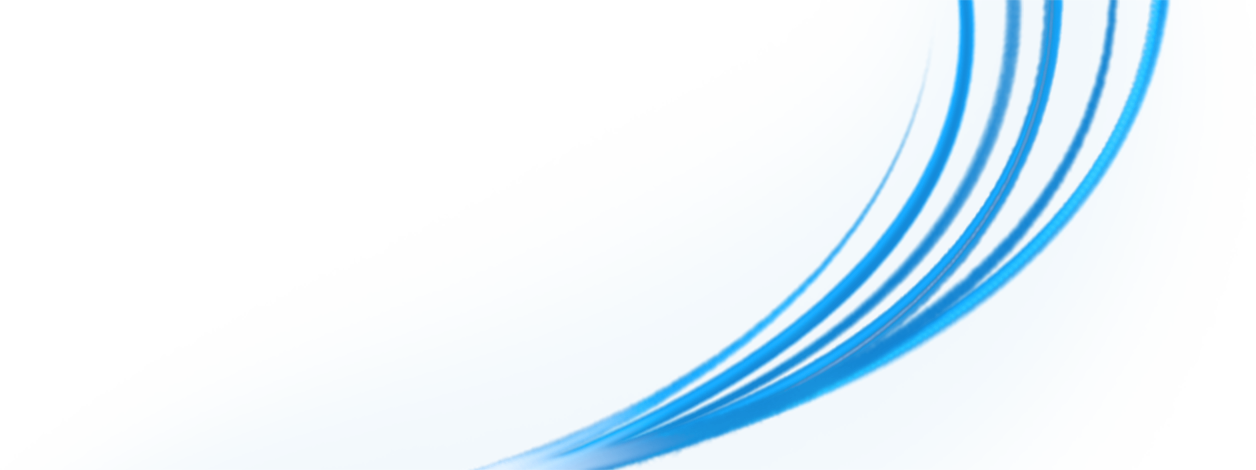
ORCA Computing and EPFL Researchers Push the Boundaries of Quantum Photonic Circuit Training for Hybrid Quantum Algorithms
Quantum computing has quickly become a driving force of innovation across multiple computational fields, from machine learning to optimization. ORCA Computing, in collaboration with researchers at the École Polytechnique Fédérale de Lausanne (EPFL), has developed a groundbreaking approach for training photonic circuits in hybrid quantum/classical algorithms. This method offers exact gradient computation, opening new possibilities and significantly enhancing the accuracy of these systems, marking a major step forward in the field.
This method diverges from prior approaches that relied on approximations of the gradients. While approximate methods may work well in scenarios where speed is the priority, ORCA’s exact method is specifically designed for tasks where every percentage of accuracy matters, for example in safety-critical applications.
Exact Gradients: The Benefits
One of the key advancements in this research is the exactness of the gradients. Previous methods for training photonic circuits used approximate gradients, which, while faster, lacked precision. This new approach offers an exact calculation of gradients, meaning that when accuracy is critical, as in certain machine learning tasks or error correction for quantum resource state generation, the results are more reliable.
Applications: Quantum Machine Learning and Beyond
One of the areas where this advancement shines is in hybrid quantum machine learning algorithms, particularly quantum neural networks. By leveraging exact gradients, the accuracy of algorithms running on ORCA’s PT Series quantum systems can be significantly improved. In a comparison between hybrid neural networks trained using this new method and the approximate “finite difference method” on a benchmark dataset, ORCA demonstrated that the new method trained faster and achieved higher accuracies than the approximate method.
The broader implications of this research extend beyond machine learning—this method can be applied to resource state generation circuits for large-scale fault-tolerant quantum computing, a vital component in realizing the long-term vision of fully scalable quantum systems.
Advancing Photonics with EPFL: A Collaborative Effort
This breakthrough in exact gradient calculation was made possible through a collaboration with researchers at EPFL, combining the expertise of both institutions to create a robust and versatile method applicable to a range of quantum computing challenges. This achievement represents a significant step forward in the training of photonic circuits, providing researchers and developers with a powerful new tool to tackle complex quantum challenges with improved precision and efficiency.